Read this blog and you’ll get: free Microsoft DP-100 exam dumps, free DP-100 exam practice tests and free DP-100 dump PDFs, and more importantly, ways to pass the Designing and Implementing a Data Science Solution on Azure exam!

Get the newest Pass4itSure DP-100 VCE dumps here: https://www.pass4itsure.com/dp-100.html (311 Q&As DP-100 Exam Dumps)
These are all things that those who want to take the DP-100 exam urgently need to know.
Microsoft DP-100 exam?
The DP-100 exam (Designing and Implementing a Data Science Solution on Azure) leads you to the Azure Data Scientist Associate certificate. Need to test your knowledge of data science and ability to implement machine learning (ML) workloads on Azure.
How to pass DP-100 exam 2022?
The PaThe Pass4itSure DP-100 exam dumps can help you maximize your exam success rate! Simply put, DP-100 exam dumps are currently the mainstream way of learning, which has been verified by countless predecessors and is very effective. No doubt! The next thing you need to do is practice.
Prep for the DP-100 exam so you pass
- Azure DP-100 practice test
- dp-100 dumps pdf google drive
Azure DP-100 exam dumps free Q&As test
Q 1
Note: This question is part of a series of questions that present the same scenario. Each question in the series contains
a unique solution that might meet the stated goals. Some question sets might have more than one correct solution,
while others might not have a correct solution.
After you answer a question in this section, you will NOT be able to return to it. As a result, these questions will not
appear in the review screen.
You are analyzing a numerical dataset that contains missing values in several columns.
You must clean the missing values using an appropriate operation without affecting the dimensionality of the feature
set.
You need to analyze a full dataset to include all values.
Solution: Remove the entire column that contains the missing data point.
Does the solution meet the goal?
A. Yes
B. No
Correct Answer: B
Use the Multiple Imputation by Chained Equations (MICE) method.
References:
https://www.ncbi.nlm.nih.gov/pmc/articles/PMC3074241/
https://docs.microsoft.com/en-us/azure/machine-learning/studio-module-reference/clean-missing-data
Q 2
HOTSPOT
You train a classification model by using a decision tree algorithm.
You create an estimator by running the following Python code. The variable feature_names is a list of all feature names,
and class_names is a list of all class names. from interpret. ext.BlackBox import TabularExplainer

You need to explain the predictions made by the model for all classes by determining the importance of all features.
For each of the following statements, select Yes if the statement is true. Otherwise, select No.
NOTE: Each correct selection is worth one point.
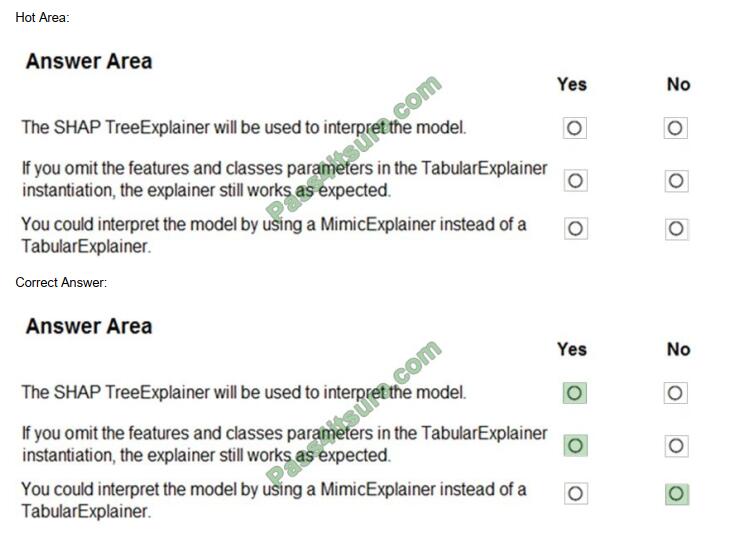
Box 1: Yes
TabularExplainer calls one of the three SHAP explainers underneath (TreeExplainer, DeepExplainer, or
KernelExplainer).
Box 2: Yes
To make your explanations and visualizations more informative, you can choose to pass in feature names and output
class names if doing classification.
Box 3: No
TabularExplainer automatically selects the most appropriate one for your use case, but you can call each of its three
underlying explainers underneath (TreeExplainer, DeepExplainer, or KernelExplainer) directly.
Reference:
https://docs.microsoft.com/en-us/azure/machine-learning/how-to-machine-learning-interpretability-aml
Q 3
You are performing feature scaling by using the sci-kit-learn Python library for x.1 x2, and x3 features. Original and
scaled data is shown in the following image.
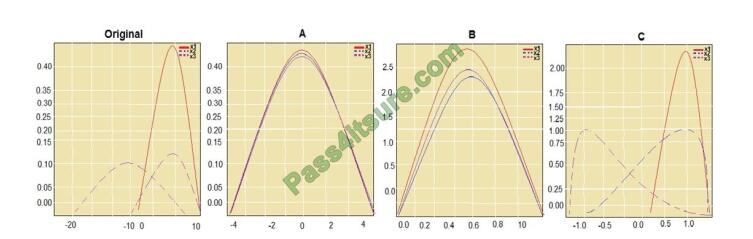
Use the drop-down menus to select the answer choice that answers each question based on the information presented
in the graphic. NOTE: Each correct selection is worth one point.
Hot Area:
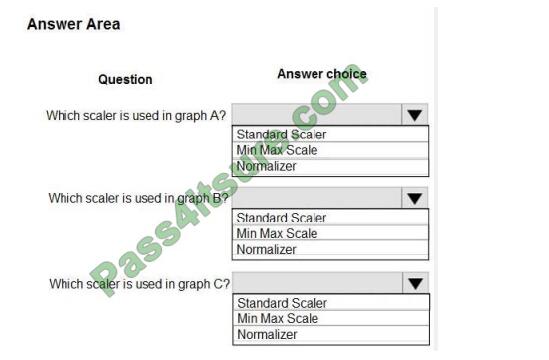
Correct Answer:
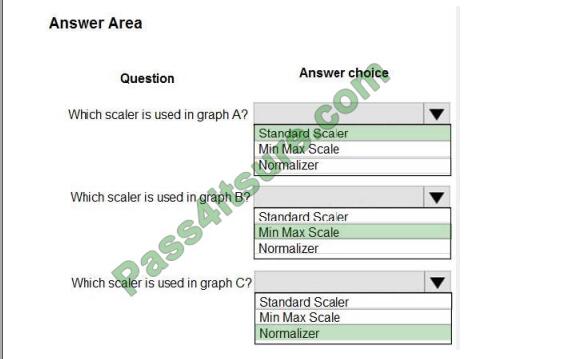
Box 1: StandardScaler
The StandardScaler assumes your data is normally distributed within each feature and will scale them such that the
distribution is now centered around 0, with a standard deviation of 1.
Example:
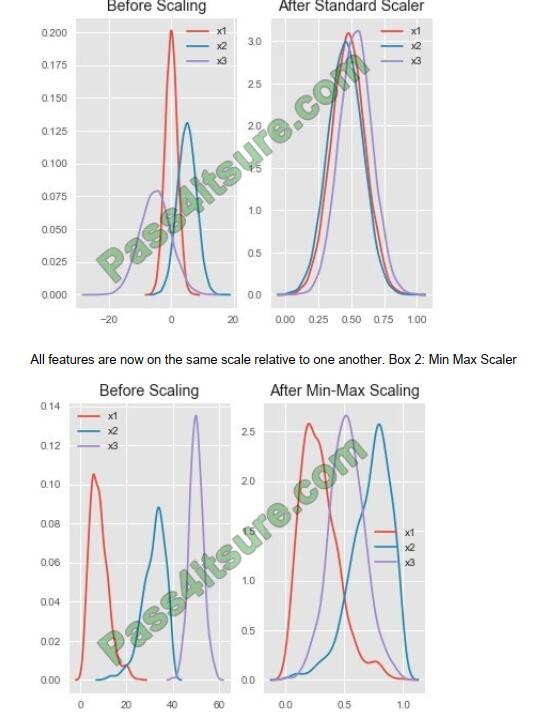
Notice that the skewness of the distribution is maintained but the 3 distributions are brought into the same scale so that they overlap.
Box 3: Normalizer
References:
http://benalexkeen.com/feature-scaling-with-scikit-learn/
Q 4
DRAG-DROP
An organization uses Azure Machine Learning services and wants to expand its use of machine learning.
You have the following compute environments. The organization does not want to create another computing
environment.
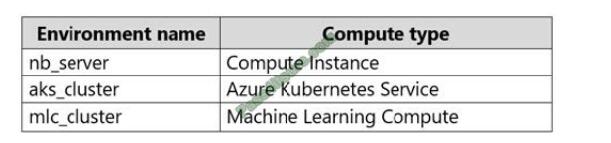
You need to determine which compute environment to use for the following scenarios.
Which compute types should you use? To answer, drag the appropriate compute environments to the correct scenarios.
Each computing environment may be used once, more than once, or not at all. You may need to drag the split bar
between panes or scroll to view content.
NOTE: Each correct selection is worth one point.
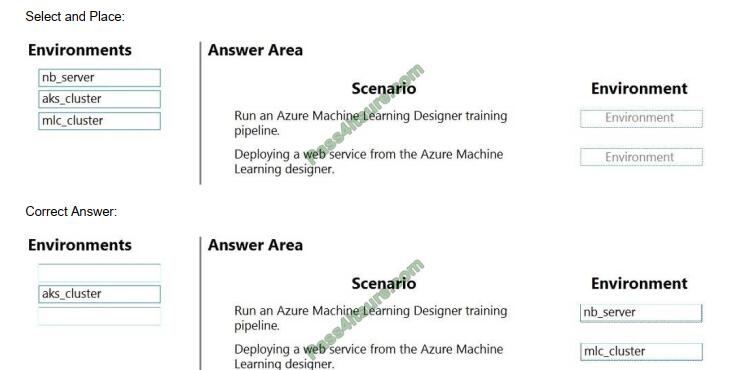
Box 1: nb_server Box 2: mlc_cluster With Azure Machine Learning, you can train your model on a variety of resources
or environments, collectively referred to as compute targets. A compute target can be a local machine or a cloud
resource, such as an Azure Machine Learning Compute, Azure HDInsight, or a remote virtual machine.
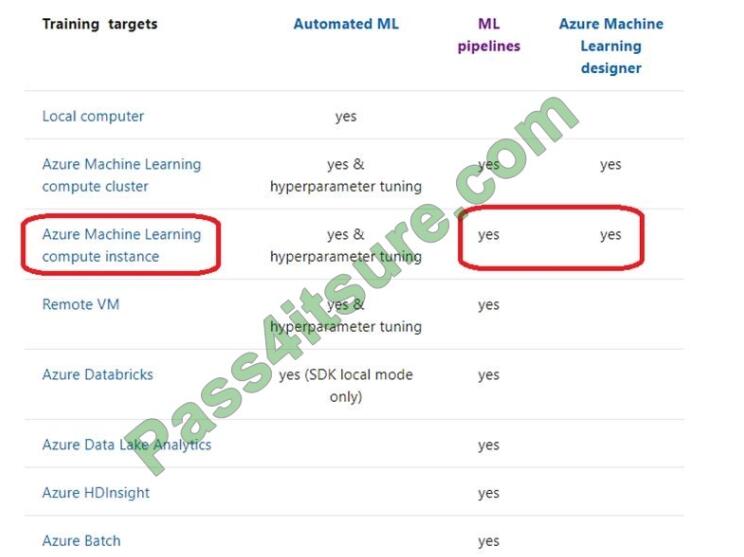
Reference:
https://docs.microsoft.com/en-us/azure/machine-learning/concept-compute-target
https://docs.microsoft.com/en-us/azure/machine-learning/how-to-set-up-training-targets
Q 5
You create a pipeline in designer to train a model that predicts automobile prices.
Because of non-linear relationships in the data, the pipeline calculates the natural log (Ln) of the prices in the training
data, trains a model to predict this natural log of price value, and then calculates the exponential of the scored label to
get the predicted price.
The training pipeline is shown in the exhibit. (Click the Training pipeline tab.)
Training pipeline
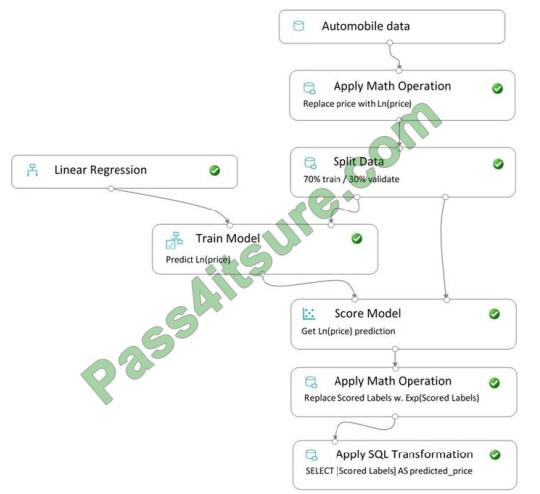
You create a real-time inference pipeline from the training pipeline, as shown in the exhibit. (Click the Real-time pipeline
tab.) Real-time pipeline
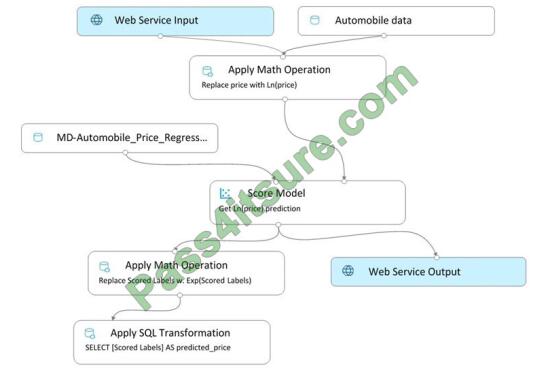
You need to modify the inference pipeline to ensure that the web service returns the exponential of the scored label as
the predicted automobile price and that client applications are not required to include a price value in the input values.
Which three modifications must you make to the inference pipeline? Each correct answer presents part of the solution.
NOTE: Each correct selection is worth one point.
A. Connect the output of the Apply SQL Transformation to the Web Service Output module.
B. Replace the Web Service Input module with a data input that does not include the price column.
C. Add a Select Columns module before the Score Model module to select all columns other than price.
D. Replace the training dataset module with a data input that does not include the price column.
E. Remove the Apply Math Operation module that replaces price with its natural log from the data flow.
F. Remove the Apply SQL Transformation module from the data flow.
Correct Answer: ACE
Q 6
You are solving a classification task.
You must evaluate your model on a limited data sample by using k-fold cross-validation. You start by configuring a k
parameter as the number of splits.
You need to configure the k parameter for the cross-validation.
Which value should you use?
A. k=0.5
B. k=0.01
C. k=5
D. k=1
Correct Answer: C
Leave One Out (LOO) cross-validation
Setting K = n (the number of observations) yields n-fold and is called leave-one-out cross-validation (LOO), a special
case of the K-fold approach.
LOO CV is sometimes useful but typically doesn\\’t shake up the data enough. The estimates from each fold are highly
correlated and hence their average can have high variance. This is why the usual choice is K=5 or 10. It provides a
good compromise for the bias-variance tradeoff.
Q 7
You use the following code to run a script as an experiment in Azure Machine Learning:
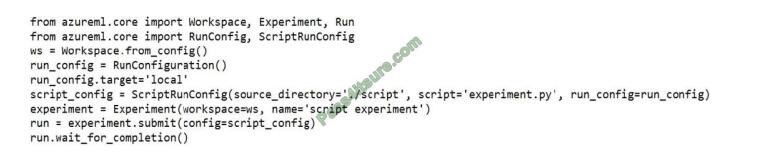
You must identify the output files that are generated by the experiment run.
You need to add code to retrieve the output file names.
Which code segment should you add to the script?
A. files = run.get_properties()
B. files= run.get_file_names()
C. files = run.get_details_with_logs()
D. files = run.get_metrics()
E. files = run.get_details()
Correct Answer: B
You can list all of the files that are associated with this run record by calling run.get_file_names()
Reference: https://docs.microsoft.com/en-us/azure/machine-learning/how-to-track-experiments
Q 8
You plan to provision an Azure Machine Learning Basic edition workspace for a data science project.
You need to identify the tasks you will be able to perform in the workspace.
Which three tasks will you be able to perform? Each correct answer presents a complete solution.
NOTE: Each correct selection is worth one point.
A. Create a Compute Instance and use it to run code in Jupyter notebooks.
B. Create an Azure Kubernetes Service (AKS) inference cluster.
C. Use the designer to train a model by dragging and dropping pre-defined modules.
D. Create a tabular dataset that supports versioning.
E. Use the Automated Machine Learning user interface to train a model.
Correct Answer: ABD
Incorrect Answers:
C, E: The UI is included in the Enterprise edition only.
Reference:
https://azure.microsoft.com/en-us/pricing/details/machine-learning/
Q 9
DRAG-DROP
You are building an experiment using the Azure Machine Learning designer.
You split a dataset into training and testing sets. You select the Two-Class Boosted Decision Tree as the algorithm.
You need to determine the Area Under the Curve (AUC) of the model.
Which three modules should you use in sequence? To answer, move the appropriate modules from the list of modules
to the answer area and arrange them in the correct order.
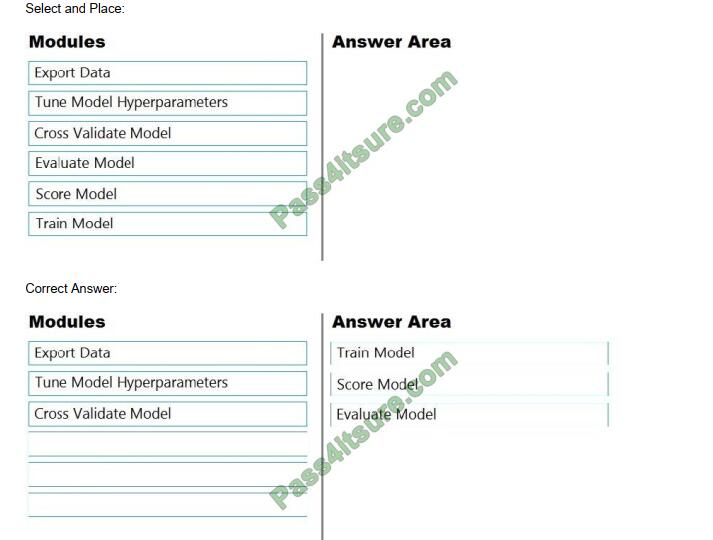
Step 1: Train Model Two-Class Boosted Decision Tree First, set up the boosted decision tree model.
1. Find the Two-Class Boosted Decision Tree module in the module palette and drag it onto the canvas.
2. Find the Train Model module, drag it onto the canvas, and then connect the output of the Two-Class Boosted Decision Tree module to the left input port of the Train Model module. The Two-Class Boosted Decision Tree module initializes the generic model, and Train Model uses training data to train the model.
3.Connect the left output of the left Execute R Script module to the right input port of the Train Model module (in this
In the tutorial you used the data coming from the left side of the Split Data module for training).
This portion of the experiment now looks something like this:
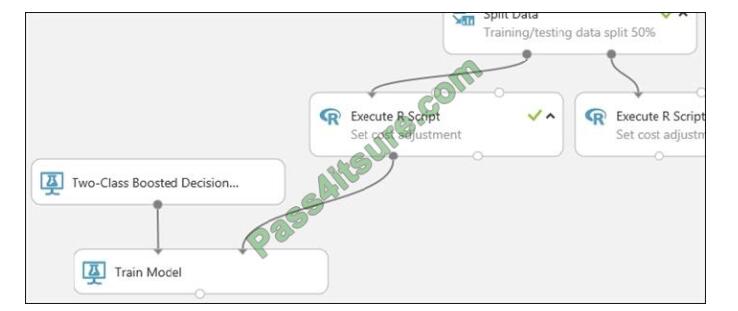
Step 2: Score Model Score and evaluate the models You use the testing data that was separated out by the Split Data
module to score our trained models. You can then compare the results of the two models to see which generated better results.
Add the Score Model modules
1.
Find the Score Model module and drag it onto the canvas.
2.
Connect the Train Model module that\\’s connected to the Two-Class Boosted Decision Tree module to the left input
port of the Score Model module.
3.
Connect the right Execute R Script module (our testing data) to the right input port of the Score Model module.
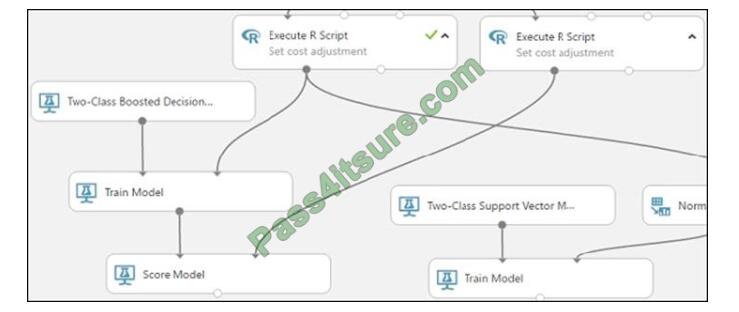
Step 3: Evaluate Model
To evaluate the two scoring results and compare them, you use an Evaluate Model module.
1.
Find the Evaluate Model module and drag it onto the canvas.
2.
Connect the output port of the Score Model module associated with the boosted decision tree model to the left input
port of the Evaluate Model module.
3.
Connect the other Score Model module to the right input port.
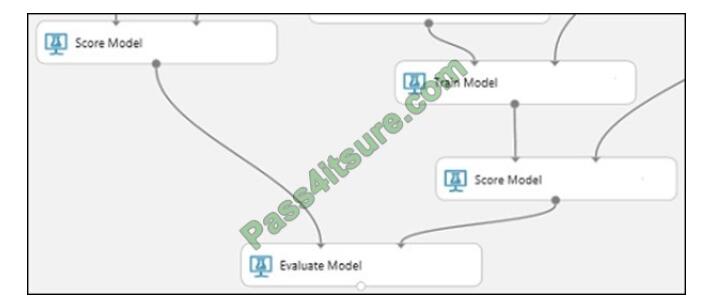
Q 10
Note: This question is part of a series of questions that present the same scenario. Each question in the series contains
a unique solution that might meet the stated goals. Some question sets might have more than one correct solution,
while others might not have a correct solution.
After you answer a question in this section, you will NOT be able to return to it. As a result, these questions will not
appear in the review screen.
You are analyzing a numerical dataset that contains missing values in several columns.
You must clean the missing values using an appropriate operation without affecting the dimensionality of the feature
set.
You need to analyze a full dataset to include all values.
Solution: Replace each missing value using the Multiple Imputation by Chained Equations (MICE) method.
Does the solution meet the goal?
A. Yes
B. No
Correct Answer: A
Replace using MICE: For each missing value, this option assigns a new value, which is calculated by using a method
described in the statistical literature as “Multivariate Imputation using Chained Equations” or “Multiple Imputation by
Chained Equations”. With a multiple imputation method, each variable with missing data is modeled conditionally using the other variables in the data before filling in the missing values.
Note: Multivariate imputation by chained equations (MICE), sometimes called “fully conditional specification” or
“sequential regression multiple imputations” has emerged in the statistical literature as one principled method of
addressing missing data. Creating multiple imputations, as opposed to single imputations, accounts for the statistical
uncertainty in the imputations. In addition, the chained equations approach is very flexible and can handle variables of
varying types (e.g., continuous or binary) as well as complexities such as bounds or survey skip patterns.
References: https://www.ncbi.nlm.nih.gov/pmc/articles/PMC3074241/
https://docs.microsoft.com/en-us/azure/machine-learning/studio-module-reference/clean-missing-data
Q 11
HOTSPOT
You write code to retrieve an experiment that is run from your Azure Machine Learning workspace.
The run used the model interpretation support in Azure Machine Learning to generate and upload a model explanation.
Business managers in your organization want to see the importance of the features in the model.
You need to print out the model features and their relative importance in an output that looks similar to the following.
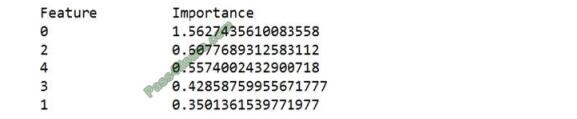
How should you complete the code? To answer, select the appropriate options in the answer area.
NOTE: Each correct selection is worth one point.
Hot Area:
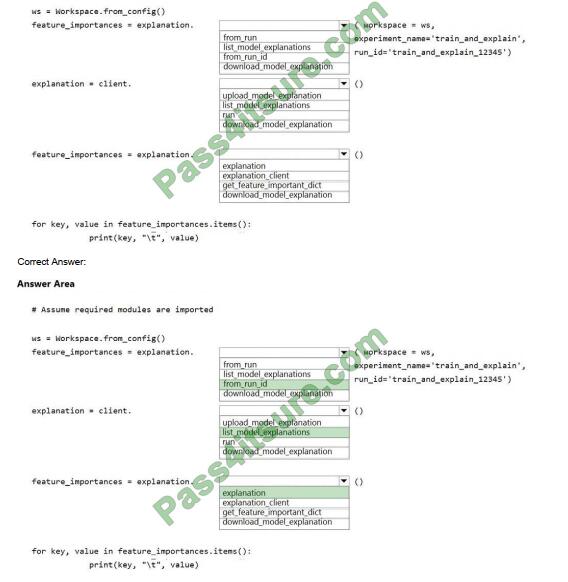
Box 1: from_run_id
from_run_id(workspace, experiment_name, run_id)
Create the client with the factory method given a run ID.
Returns an instance of the ExplanationClient.
Parameters Workspace An object that represents a workspace.
experiment_name str The name of an experiment.
run_id str A GUID that represents a run.
Box 2: list_model_explanations
list_model_explanations returns a dictionary of metadata for all model explanations available.
Returns A dictionary of metadata such as id, data type, method, model type, and upload time, sorted by upload time
Box 3:
Q 12
HOTSPOT
You need to identify the methods for dividing the data according, to the testing requirements.
Which properties should you select? To answer, select the appropriate option-, m the answer area;
NOTE: Each correct selection is worth one point.
Hot Area:
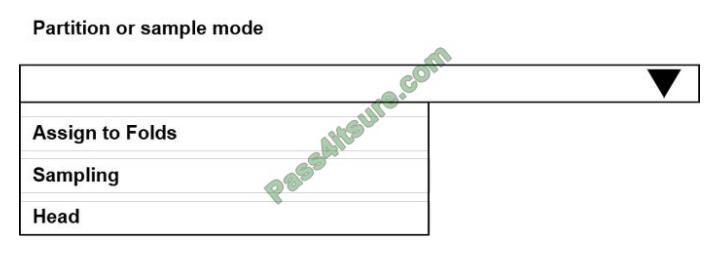
Correct Answer:
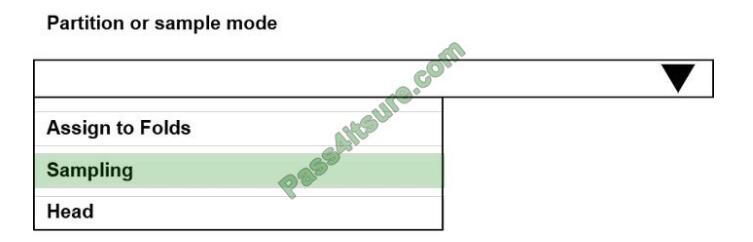
Take the above MB-330 exam test to verify your strength online.
DP-100 dumps pdf google drive download
DP-100 exam pdf free https://drive.google.com/file/d/1gKitMnepKD4M9K35tngA9RJq-1U1uJeK/view?usp=sharing
Pass4itSure shares a valid DP-100 exam dumps 2022 to help pass the DP-100 exam! Pass4itSure now offers the latest DP-100 VCE dumps and DP-100 PDF dumps, Pass4itSure DP-100 exam question 2022 has been updated and corrected answers, please get the latest Pass4itSure DP-100 dumps with VCE and PDF here: https://www.pass4itsure.com/dp-100.html (311 Q&A dumps).